Research interests
Our research is dedicated to developing computational algorithms and conducting large-scale data mining from single-cell multiomic data. We aim to unravel the intricate mechanisms of gene regulation, cellular crosstalk, and metabolic reprogramming that define cell identities. Additionally, we have created methods and web resources specifically designed for analyzing single-cell multi-omics, spatial multi-omics, single-cell CRISPR screen data, performing signal enhancement, and multimodal translation. By seamlessly integrating single-cell datasets with spatial and multimodal information, our goal is to establish quantitative connections between changes in cell identity and disease phenotypes in various biological systems, including cancer, development, and aging.
Intelligent Algorithms for Enhancing, Generating, and Functionally Analyzing Single-Cell Spatial Multi-Omics
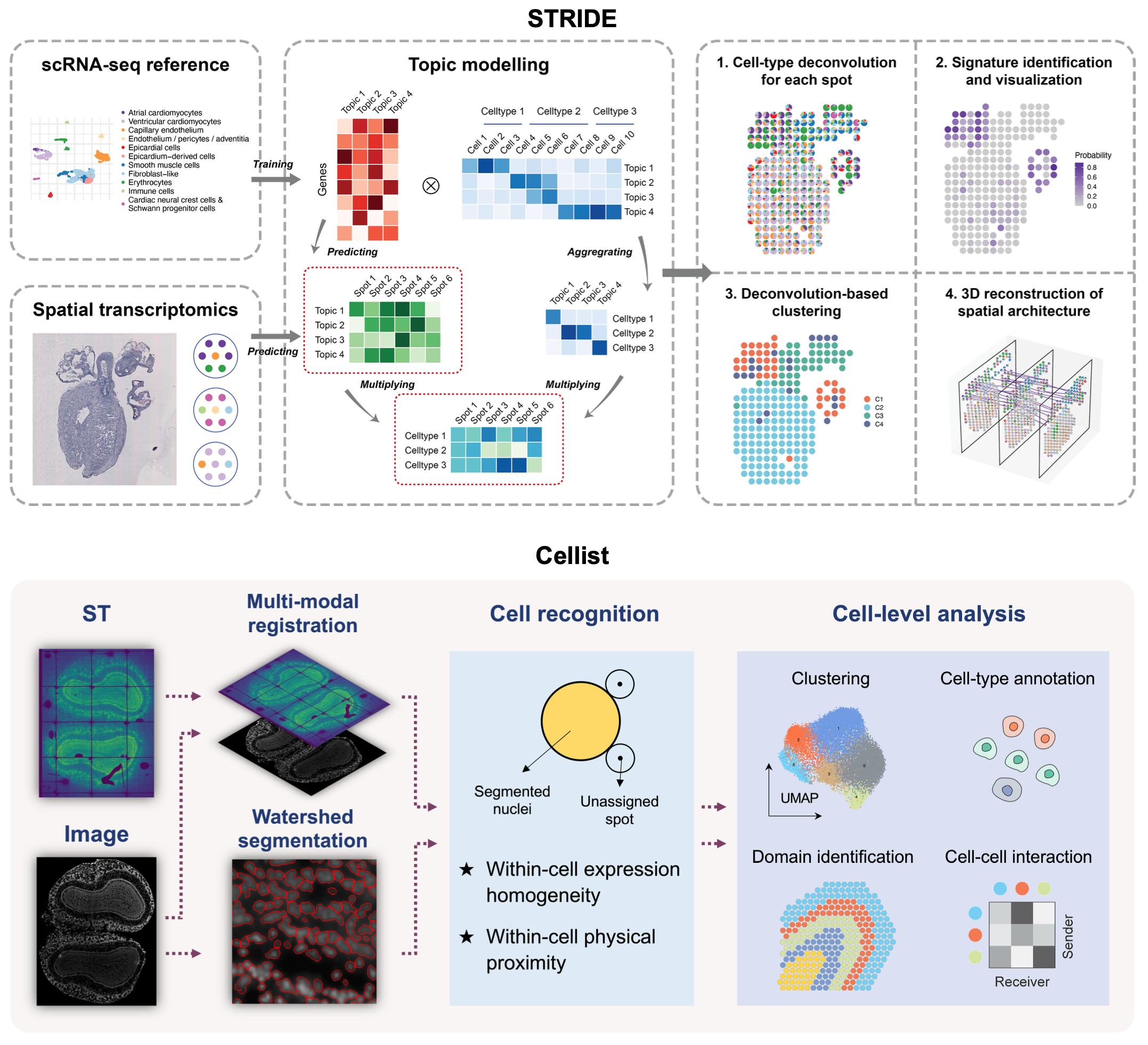
Single-cell and spatial multi-omic technologies have revolutionized our understanding of cellular heterogeneity in complex biological systems. However, corresponding analyses currently face various challenges, including inadequate resolution, coverage, and difficulty integrating and generating heterogeneous multi-modal data. To address these issues, we have developed a series of intelligent algorithms. STRIDE and Cellist enhance low-resolution and high-resolution spatial transcriptomics data signals, respectively, elevating them to the single-cell level. SCRIP and SCRIPro constructs gene regulation networks using single-cell and spatial multiomics data. EvaCCI assesses intercellular interactions , while SCREE analyzes multimodal single-cell CRISPR screen data. These algorithms enhance the usability of single-cell spatial omics data and lay the foundation for the integrative analysis of spatiotemporal multimodal data, as well as computational modeling of cellular phenotypes in physiological and pathological conditions.
Cell Phenotype Modeling Based on Generative AI and Large-Scale Spatiotemporal Multimodal Data
Cell phenotypes in multicellular systems are regulated by intrinsic factors (such as gene expression regulation) and extrinsic factors (such as intercellular interactions). We have demonstrated the tight connection between intrinsic epigenetic regulations and cell fate determination in mouse IVF and SCNT embryo development (Nature, 2016; Nat. Cell Biol., 2018; Cell Stem Cell, 2018, 2022; Cell Res., 2022). Currently, we are developing generative AI models that are pretrained on large-scale single-cell multi-modal datasets. These models aim to uncover the collaborative effects between gene regulation, cellular crosstalk, and other environmental factors, such as metabolites and mechanics, on cell phenotypes. As a pioneering work, we have developed SELINA, which utilizes a multi-adversarial domain adaptation network to automatically annotate cell types using a large-scale pretrained human scRNA-seq reference. We hope to leverage generative AI models to gain deeper insights into the molecular mechanisms driving cell phenotypes and further guide and reshape this transformation process.
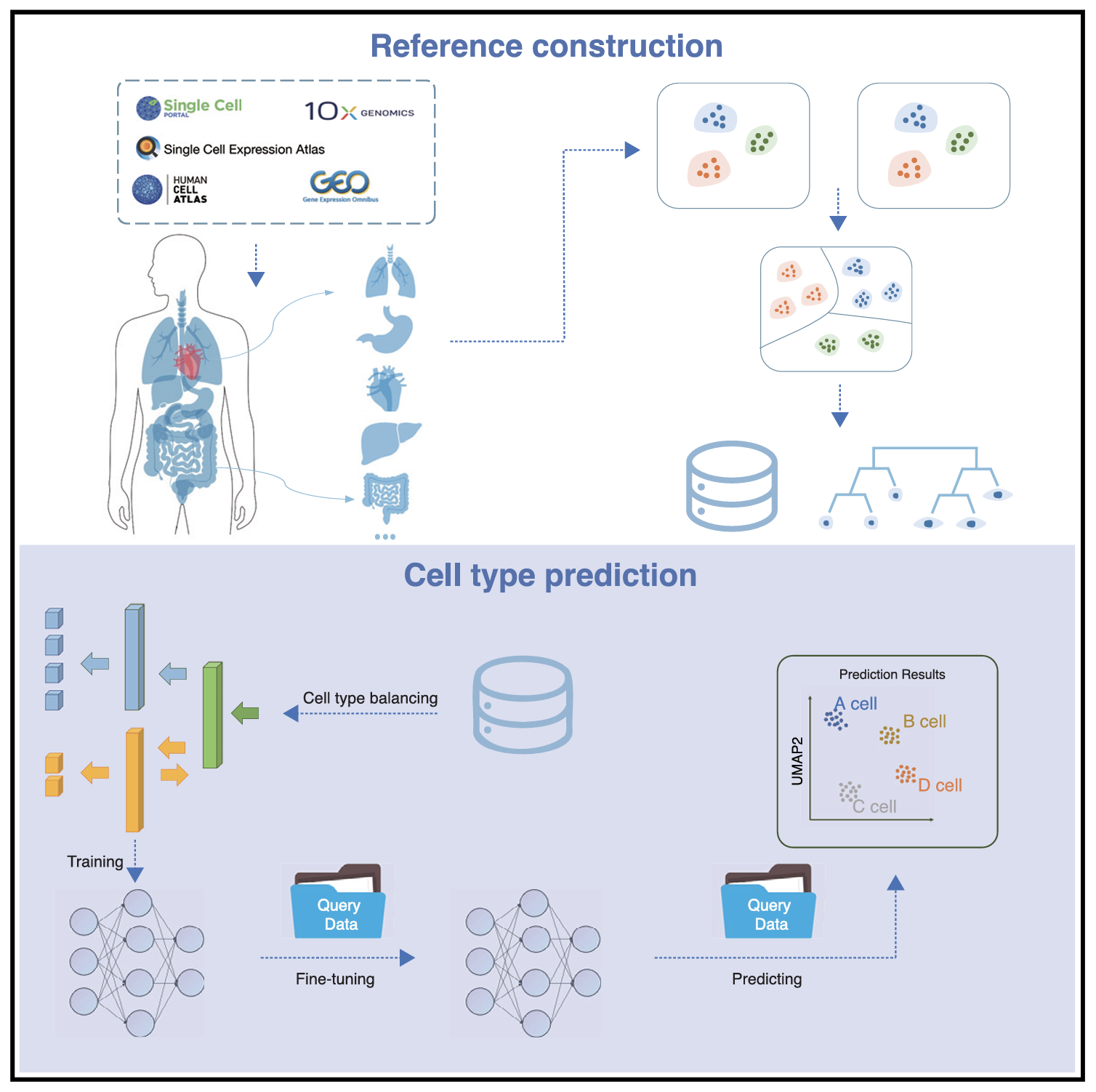
Diversity, Plasticity, and Association of Cell Phenotypes to Individual Phenotypes in Complex Disease Microenvironments
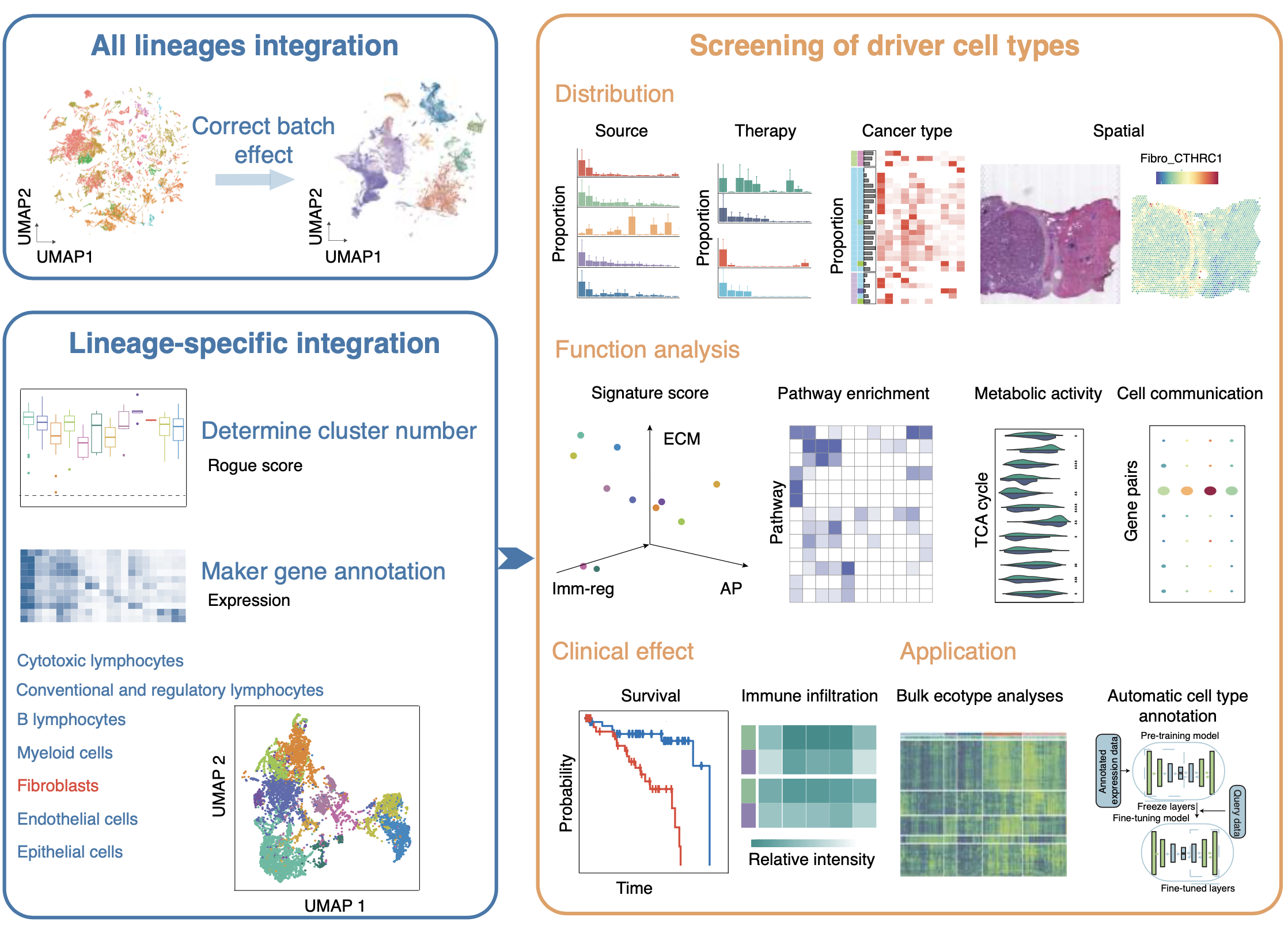
Cancer results from immune surveillance evasion, with the immunosuppressive tumor microenvironment having a profound impact on tumor development and treatment resistance. Our research team combines deep bioinformatics data analysis with single-cell spatiotemporal multi-omics data to study the effects of intrinsic gene expression regulation and extrinsic environmental factors on disease-related cell phenotypes in the tumor microenvironment, as well as the association between cell phenotypes and individual phenotypes. We have established a comprehensive single-cell RNA sequencing data resource, TISCH, for analyzing gene expression and cellular composition in the tumor microenvironment. We have also constructed a cell phenotype atlas for all cancer types, TabulaTIME ), and discovered a widely prevalent profibrotic ecotype that regulates tumor immunity. Our team is currently collaborating closely with oncologists and immunologists to investigate the mechanisms of tumor microenvironment evolution and immune therapy resistance in different cancer types (Cell 2024; Nat. Genet. 2024; Cancer Immunol. Res. 2023; Genome Med. 2023; EMBO J. 2023).